|
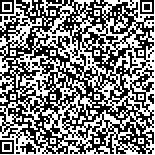 二维码(扫一下试试看!) |
基于机器学习的箱纸板质量预测模型构建及比较 |
Construction and Comparison of Cardboard Quality Prediction Models Based on Machine Learning |
收稿日期:2022-08-11 |
DOI:10.11980/j.issn.0254-508X.2023.07.009 |
关键词: 机器学习 数据挖掘 造纸工业 质量预测 软测量 |
Key Words:machine learning data mining paper industry quality prediction soft measurement |
基金项目:国家重点研发计划(2020YFE0201400);人工智能与数字经济广东省实验室(广州)青年学者项目(PLZ2021KF0019)。 |
|
摘要点击次数: 2130 |
全文下载次数: 1090 |
摘要:箱纸板生产涉及一系列复杂工艺流程,且由于缺乏关键质量的在线监测手段,进而导致质量管控困难。为此,本研究尝试基于机器学习方法建立可在线监测箱纸板质量的预测模型,也称软测量模型,以促进上述问题的有效解决。本研究采用箱纸板企业实际数据,训练并比较了随机森林(RF)、梯度提升回归(GBR)、K近邻回归(KNN)及偏最小二乘回归(PLS)在多项质量指标上的预测表现。结果表明,不同质量指标本身很大程度上影响了预测精度的上限,而不同算法对理论上限的逼近程度有显著差异。复杂、非线性的集成模型(RF、GBR)相较于简单模型(KNN、PLS)有更好的表现。 |
Abstract:The production of cardboard in volves a series of complex processes and the lack of online monitoring methods for key qualities, which makes it difficult to control the quality of cardboard. This paper attempted to establish predictive models, also known as soft measurement models, which based on machine learning methods that could monitor cardboard quality on line to facilitate effective solutions to the above problems. This study used actual data from cardboard companies to train and compared the predictive performance of random forest (RF), gradient boosted regression (GBR), K-nearest neighbor regression (KNN), and partial least squares regression (PLS) on a variety of quality indicators. The results showed that the different quality indicators themselves largely effected the upper limit of prediction accuracy, while the degree of approximation to the theoretical upper limit varied significantly among algorithms. Complex, nonlinear integrated models (RF, GBR) had better performance, compared to simple models (KNN,PLS). |
查看全文 HTML 查看/发表评论 下载PDF阅读器 |