|
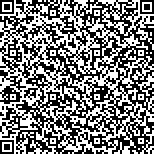 二维码(扫一下试试看!) |
基于RPLS的造纸废水处理过程软测量建模 |
Soft Sensor Modeling of Papermaking Effluent Treatment Processes Using RPLS |
收稿日期: |
DOI:10.11980/j.issn.0254-508X.2016.10.007 |
关键词: 递归偏最小二乘 偏最小二乘 软测量建模 造纸废水处理 |
Key Words:recursive partial least squares partial least squares soft sensor modeling papermaking effluent treatment |
基金项目:南京林业大学高层次人才科研启动基金(No.16310-5996);江苏省制浆造纸科学与技术重点实验室开放基金项目(201010);江苏高校优势学科建设工程资助项目(PAPD)。 |
作者 | 单位 | 杨 浩1 | 1.南京林业大学江苏省制浆造纸科学与技术重点实验室,江苏南京,210037 | 莫卫林1 | 1.南京林业大学江苏省制浆造纸科学与技术重点实验室,江苏南京,210037 | 熊智新1 | 1.南京林业大学江苏省制浆造纸科学与技术重点实验室,江苏南京,210037 | 黄明智2 | 2.中山大学水资源与环境系,广东广州,510275 | 刘鸿斌1,* | 1.南京林业大学江苏省制浆造纸科学与技术重点实验室,江苏南京,210037 |
|
摘要点击次数: 6307 |
全文下载次数: 2137 |
摘要:偏最小二乘(PLS)软测量预测模型在预测造纸废水处理过程中的出水化学需氧量(CODCr)和固体悬浮物(SS)时,易受过程非线性特性和系统外部干扰等因素的影响而失效。针对以上问题,研究了递归偏最小二乘(RPLS)算法的造纸废水处理过程软测量建模。计算结果表明,采用PLS模型预测出水CODCr时,平均绝对百分比误差(MAPE)、均方根误差(RMSE)和相关系数(R2)分别为5.3832%、4.6878和0.5892;采用RPLS模型预测时,MAPE、RMSE、R2分别为1.3861%、1.8792和0.9221。采用PLS模型预测SS时,MAPE、RMSE和R2分别为2.5962%、0.7412和0.6651;采用RPLS模型时MAPE、RMSE、R2分别为0.6795%、0.2198和0.9627。以上结果表明,RPLS预测模型比PLS预测模型具有更好的预测性能和更高的精度。 |
Abstract:Soft sensor modeling methods based on partial least squares (PLS) and recursive PLS (RPLS)were used to predict effluent chemical oxygen demand(CODCr) and effluent suspended solids(SS) in a papermaking wastewater treatment process. PLS is unsuitable for the systems with non-linear characteristics and external disturbances. The results showed that the mean absolute percentage error(MAPE),root mean square error(RMSE), and squared correlation coefficient(R2) for CODCr using PLS were 5.3832%, 4.6878, and 0.5892, respectively, and they were 1.3861%, 1.8792, and 0.9221, respectively using RPLS. In terms of SS, the MAPE, RMSE, and R2 were 2.5962%,0.7412, and 0.6651, respectively when using PLS, and the three indices using RPLS were 0.6795%, 0.2198, and 0.9627, respectively. These results indicated that the RPLS model had better prediction performance and higher accuracy compared to the PLS model. |
查看全文 HTML 查看/发表评论 下载PDF阅读器 |
|
|
|